Brain–Computer Interface Experiments First to Decode Words 'Spoken' Entirely In the Brain In Real Timehttps://medicalxpress.com/news/2024-05-braincomputer-interface-decode-words-spoken.htmlhttps://techxplore.com/news/2024-05-brain-machine-interface-device-internal.htmlCaltech neuroscientists are making promising progress toward showing that a device known as a brain–machine interface (BMI), which they developed to implant into the brains of patients who have lost the ability to speak, could one day help all such patients communicate by simply thinking and not speaking or miming.In 2022, the team reported that their BMI had been successfully implanted and used by a patient to communicate unspoken words. Now, reporting in the journal
Nature Human Behaviour, the scientists have shown that the BMI has worked successfully in a second human patient.
BMIs are being developed and tested to help patients in a number of ways. For example, some work has focused on developing BMIs that can control robotic arms or hands. Other groups have had success at predicting participants' speech by analyzing brain signals recorded from motor areas when a participant whispered or mimed words.
But predicting what somebody is thinking—detecting their internal dialogue—is much more difficult, as it does not involve any movement, explains Sarah Wandelt, Ph.D., lead author on the new paper, who is now a neural engineer at the Feinstein Institutes for Medical Research in Manhasset, New York.
... "We reproduced the results in a second individual, which means that this is not dependent on the particulars of one person's brain or where exactly their implant landed. This is indeed more likely to hold up in the larger population."
The new research is the most accurate yet at predicting internal words. In this case, brain signals were recorded from single neurons in a brain area called the supramarginal gyrus located in the posterior parietal cortex (PPC). The researchers had found in a previous study that this brain area represents spoken words.
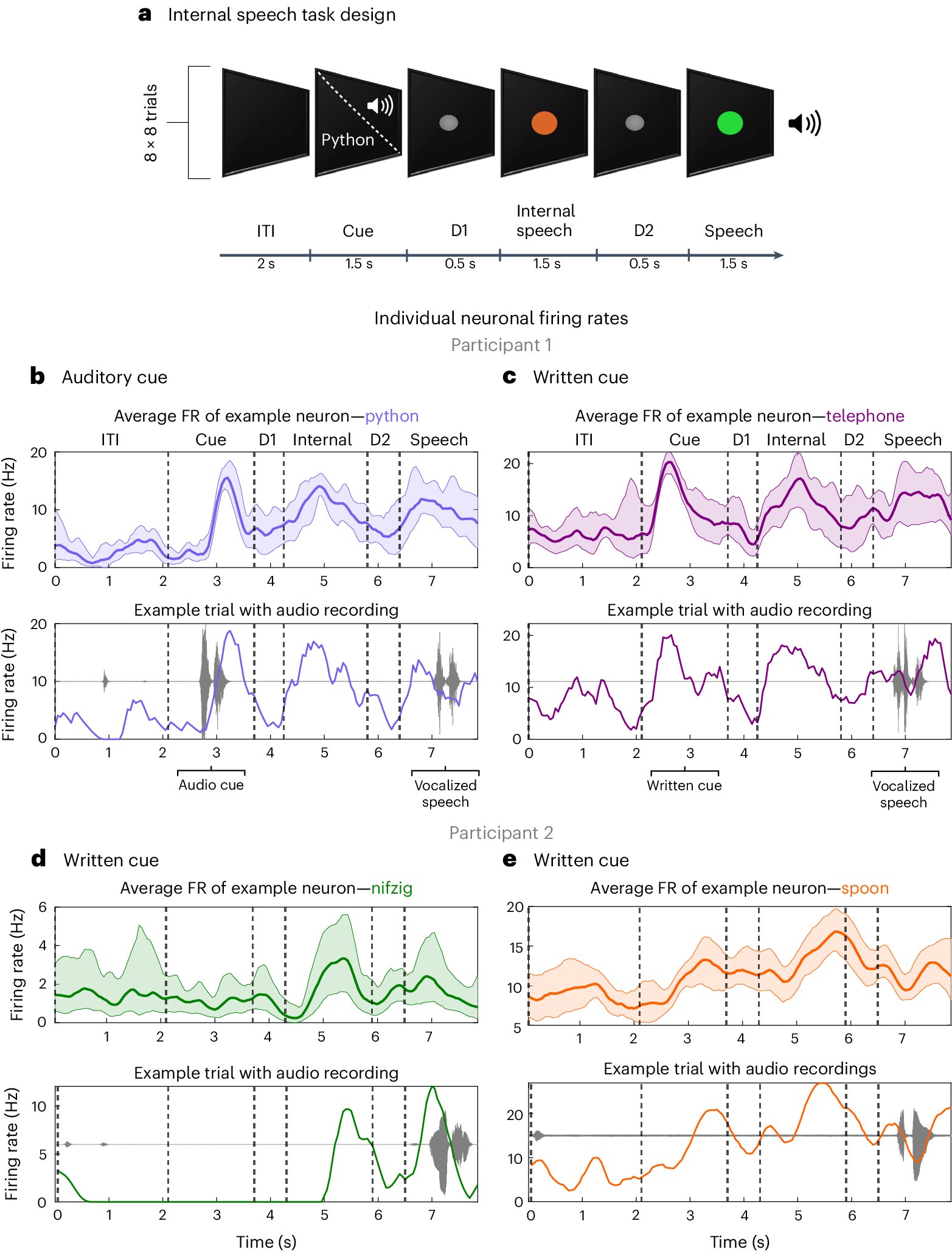
In the current study, the researchers first trained the BMI device to recognize the brain patterns produced when certain words were spoken internally, or thought, by two tetraplegic participants. This training period took only about 15 minutes. The researchers then flashed a word on a screen and asked the participant to "say" the word internally. The results showed that the BMI algorithms were able to predict the eight words tested, including two nonsensical words, with an average of 79% and 23% accuracy for the two participants, respectively.
Words can be significantly decoded during internal speech in the SMG."Since we were able to find these signals in this particular brain region, the PPC, in a second participant, we can now be sure that this area contains these speech signals," says David Bjanes, a postdoctoral scholar research associate in biology and biological engineering and an author of the new paper. "The PPC encodes a large variety of different task variables. You could imagine that some words could be tied to other variables in the brain for one person. The likelihood of that being true for two people is much, much lower."
Sarah K. Wandelt et al,
Representation of internal speech by single neurons in human supramarginal gyrus,
Nature Human Behaviour (2024)
https://www.nature.com/articles/s41562-024-01867-yBrain–machine-interface device translates internal speech into text,
Nature Human Behaviour (2024)
https://www.nature.com/articles/s41562-024-01869-w---------------------------------------------------------------
Brain Signals Transformed Into Speech Through Implants and AIhttps://medicalxpress.com/news/2023-08-brain-speech-implants-ai.htmlResearchers from Radboud University and the UMC Utrecht have succeeded in transforming brain signals into audible speech. By decoding signals from the brain through a combination of implants and AI, they were able to predict the words people wanted to say with an accuracy of 92 to 100%. Their findings are published in the
Journal of Neural Engineering.
... For the experiment in their new paper, the researchers asked non-paralyzed people with temporary brain implants to speak a number of words out loud while their brain activity was being measured.
Berezutskaya says, "We were then able to establish direct mapping between brain activity on the one hand, and speech on the other hand. We also used advanced artificial intelligence models to translate that brain activity directly into audible speech. That means we weren't just able to guess what people were saying, but we could immediately transform those words into intelligible, understandable sounds. In addition, the reconstructed speech even sounded like the original speaker in their tone of voice and manner of speaking."
Julia Berezutskaya et al,
Direct speech reconstruction from sensorimotor brain activity with optimized deep learning models,
Journal of Neural Engineering (2023)
https://iopscience.iop.org/article/10.1088/1741-2552/ace8be---------------------------------------------------------------
Refined AI Approach Improves Noninvasive Brain-Computer Interface Performancehttps://techxplore.com/news/2024-05-refined-ai-approach-noninvasive-brain.htmlPursuing a viable alternative to invasive brain-computer interfaces (BCIs) has been a continued research focus of Carnegie Mellon University's He Lab. In 2019, the group used a noninvasive BCI to successfully demonstrate, for the first time, that a mind-controlled robotic arm had the ability to continuously track and follow a computer cursor.As technology has improved, their AI-powered deep learning approach has become more robust and effective. In new work published in
PNAS Nexus, the group demonstrates that humans can control continuous tracking of a moving object all by thinking about it, with unmatched performance.
... In a recent study by Bin He, professor of biomedical engineering at Carnegie Mellon University, a group of 28 human participants were given a complex BCI task to track an object in a two-dimensional space all by thinking about it.
During the task, an electroencephalography (EEG) method recorded their activity, from outside the brain. Using AI to train a deep neural network, the He group then directly decoded and interpreted human intentions for continuous object movement using the BCI sensor data.
Overall, the work demonstrates the excellent performance of noninvasive BCI for a brain-controlled computerized device.
"The innovation in AI technology has enabled us to greatly improve the performance versus conventional techniques, and shed light for wide human application in the future," said Bin He.
Moreover, the capability of the group's AI-powered BCI suggests a direct application to continuously controlling a robotic device.
"We are currently testing this AI-powered noninvasive BCI technology to control sophisticated tasks of a robotic arm," said He. "Also, we are further testing its applicability to not only able-body subjects, but also stroke patients suffering motor impairments."
In a few years, this may lead to AI-powered assistive robots becoming available to a broad range of potential users.
Dylan Forenzo et al,
Continuous tracking using deep learning-based decoding for noninvasive brain–computer interface,
PNAS Nexus (2024)
https://academic.oup.com/pnasnexus/article/3/4/pgae145/7656016?login=false-------------------------------------------------------
Decoding Spontaneous Thoughts From the Brain via Machine Learninghttps://medicalxpress.com/news/2024-04-decoding-spontaneous-thoughts-brain-machine.html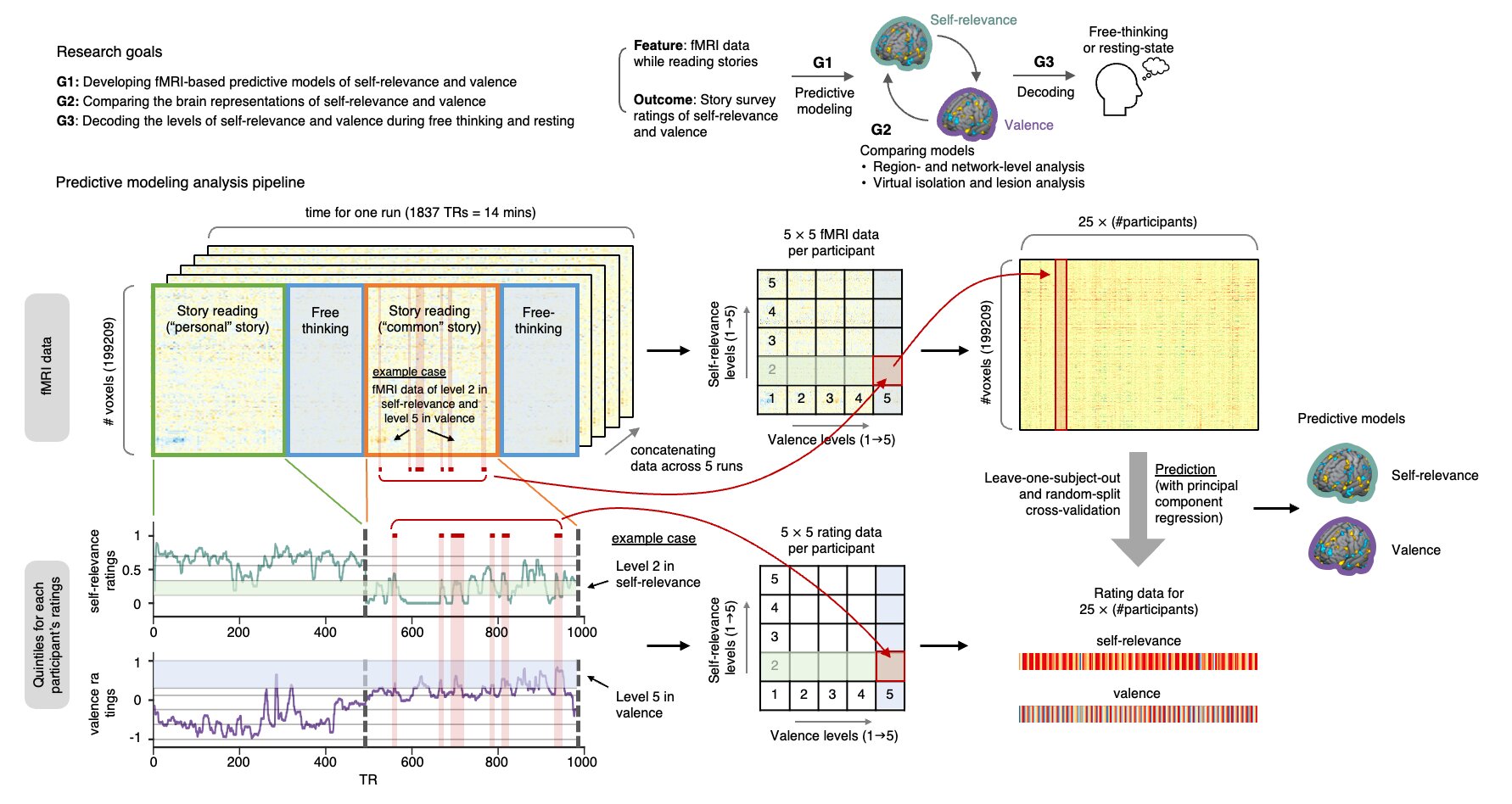
Researchers demonstrated the possibility of using functional magnetic resonance imaging (fMRI) and machine learning algorithms to predict subjective feelings in people's thoughts while reading stories or in a freely thinking state. The study is published in the Proceedings of the National Academy of Sciences.
... New research suggests that it may be possible to develop predictive models of affective contents during spontaneous thought by combining personal narratives with fMRI. Narratives and spontaneous thoughts share similar characteristics, including rich semantic information and temporally unfolding nature. To capture a diverse range of thought patterns, participants engaged in one-on-one interviews to craft personalized narrative stimuli, reflecting their past experiences and emotions. While participants read their stories inside the MRI scanner, their brain activity was recorded.
Hong Ji Kim et al,
Brain decoding of spontaneous thought: Predictive modeling of self-relevance and valence using personal narratives,
Proceedings of the National Academy of Sciences (2024)
https://www.pnas.org/doi/10.1073/pnas.2401959121-------------------------------------------------------
Wearable Devices Can Now Harvest Neural Data—Urgent Privacy Reforms Neededhttps://techxplore.com/news/2024-05-wearable-devices-harvest-neural-urgent.htmlRecent trends show Australians are increasingly buying wearables such as smartwatches and fitness trackers. These electronics track our body movements or vital signs to provide data throughout the day, with or without the help of artificial intelligence (AI).
There's also a newer product category that engages directly with the brain. It's part of what UNESCO broadly defines as the emerging industry of "neurotechnology": "devices and procedures that seek to access, assess, emulate and act on neural systems."Much of neurotechnology is either still in development stage, or confined to research and medical settings. But consumers can already purchase several headsets that use electroencephalography (EEG).
Often marketed as meditation headbands, these devices provide real-time data on a person's brain activity and feed it into an app.Such headsets can be useful for people wanting to meditate, monitor their sleep and improve wellness. However, they also raise privacy concerns—a person's brain activity is intrinsically personal data.
The subtle creep in neural and cognitive data wearables are capable of collecting is resulting in a data "gold rush," with companies mining even our brains so they can develop and improve their products.... The private data collected through such devices is increasingly fed into AI algorithms, raising additional concerns. These algorithms rely on machine learning, which can manipulate datasets in ways unlikely to align with any consent given by a user.
... Australia is at a pivotal crossroads. We need to address the risks associated with data harvesting through neurotechnology. The industry of devices that can access our neural and cognitive data is only going to expand.
-----------------------------------------------------